Sunday, February 28, 2010
Deinterlacing
Video Analytics Survey
- Video Analytics: Business Intelligence + Security
Take the international clothing retailer Benetton, which is using video analytics to glean intelligence about customer shopping habits, potentially lucrative information. Yet when enterprises do discuss the strategic benefits of analytics beyond security uses, they often come up in the second round of talking points. This is unfortunate because that strategic focus, the market is getting bogged down in a debate about architecture, particularly whether edge-based or server-based analytics are superior. More in
4. The Rise of Video Analytics
By Josh Manion
Chief Executive Officer
Stratigent, LLC
According to eMarketer, online video advertisements grew 89% from 2006 to 2007, and are projected to grow 68% from 2007 to 2008 to $1.3 billion dollars. By 2010, online video advertising is expected to reach almost $3 billion. And by 2011, almost 90% of the U.S. internet population will have consumed online video.
What is behind the rise of online video?
Clearly, the acquisition of YouTube by Google broadened the reach of online video and encouraged active participation by users. The availability of news, movies and TV shows online is also driving the growth of online video. NBC expects 2,200 hours of Olympic events to be online and available for viewing. Also, the social engagement of easily sharing videos with others is driving growth.
Making video analytics actionable
Despite this significant growth in the adoption of online video, effectively measuring and analyzing the usage of online video is still in its infancy. Many issues must be overcome including technical challenges to the many different video player formats currently being used, uncertainly about the most useful KPI’s, and the overall lack of talented analytics resources that have experience with video analytics.
In order to start taking action on video analytics it is helpful to first outline a high-level grouping of the types of metrics (or video analytics KPI’s) that I have found to be of interest when it comes to measuring video analytics.
- Basic Metrics: This includes views, visits, unique viewers, duration of views, and the technical profiles of the visitors, etc.
- Engagement: Which videos have the highest visitor engagement, where do visitors abandon the video, and which video segments capture their attention and cause them to rewind, etc. Applying this type of data to the specific video asset in question is often a key requirement.
- Distribution: Distribution helps to understand where video’s are consumed, URL’s they are being viewed at, the type of referral source, (e.g. email, RSS, etc) and geography of the viewers consuming content.
The next step in the process is to select an appropriate set of technology to collect and analyze video analytics data. Several tools provide varying degrees of video measurement. Traditional web analytics vendors like Omniture and WebTrends both released video analytics functionality this year. Another option is to work with firms that specialize in video analytics, such as Visible Measures. In each case, you will have the capability to track the basic metrics, but in the case of the more specialized firms you will have access to additional capabilities around tracking distribution, engagement and the performance of individual video assets within the context of the video itself. As always, when considering this type of investment, it is important to consider the level of effort required for the implementation, which is often dependant on the number and type of video players your organization uses. Additionally, I encourage you to consider the level of data integration needed with your other marketing analytics data so you can effectively evaluate the total investment necessary to meet your business requirements.
For more information please contact 877-427-8900 or email info@stratigent.com.
5. Top 3 Problems Limiting the Use and Growth of Video Analytics
http://ipvideomarket.info/report/top_3_problems_limiting_the_use_and_growth_of_video_analytics
by John Honovich, IP Video Market Info posted on Jun 19, 2008
While video analytics holds great promise, people are still asking about the viability of using analytics in the real world. Indeed, as stories of video analytic problems have spread, concerns about the risks of video analytics now seem higher than a few years ago when the novelty of the technology spurred wide excitement.
This article surveys the main problems limiting the use and growth of video analytics. It is meant to help security managers and integrators gain a better sense of the core issues involved.
Top 3 Problems:
- Eliminating False Alerts
- System Maintenance Too Difficult
- Cost of System Too High
Eliminating False Alerts
Since the goal of video analytics is to eliminate human involvement, eliminating false alerts is necessary to accomplish this. Each false alerts not only requires a human assessment, it increases emotional and organizational frustration with the system.
Most are familiar with burglar alarm false alarms and the frustration these causes. On average, burglar alarm false alarm per house or business are fairly rare. If you have 1 or 2 per month, that is fairly high. Many people do not experience false alarms of their burglar system for months.
By contrast, many video analytic systems can generate dozens of false alarms per day. This creates a far greater issue than anything one is accustomed to with burglar alarms. Plus, with such alarms happening many times throughout the day, it can become an operational burden.
Now, not all video analytics systems generate lots of false alarms but many do. These issues have been the number one issue limitation of the integrators and end-users that I know using and trying video analytics.
System Maintenance Too Difficult
System maintenance is a often overlooked and somewhat hidden issue in video analytics.
Over a period of weeks or months, a video analytic system's false alerts can start rising considerably due to changes in the environment, weather and the position of the sun. This can suddenly and surprisingly cause major problems with the system.
Not only is the increase in false alerts a problem, the risk now that the system could unexpectedly break in the future creates a significant problem in trust. If your perimeter surveillance one day stops functioning properly, you now have a serious flaw in your overall security plan.
This has been a cause of a number of video analytic system failures. The systems, already purchased, simply get put to the side becoming a very expensive testament to not buying or referring one's colleagues to video analytics.
This being said, not all video analytic systems exhibit this behavior but you would be prudent to carefully check references to verify that existing systems have been operating for a long period of time without any major degradation.
Cost of System Too High
While you can find inexpensive video analytic systems today, these system tend to exhibit problems 1 and 2, high false alerts and poor system maintenance. Indeed, in my experience, video analytic systems that are either free or only cost $100-$200 more generally have significant operational problems.
One common feature of systems that work is that the complete price for hardware and software is usually $500 or more per channel for the analytics. Now just because a video analytic systems is expensive obviously does not mean it is good. However, there are necessary costs in building a systems that is robust and works well in the real world.
The cost of video analytic systems comes in making them robust to real world conditions that we all take for granted. The developer needs to make the video analytic system “intelligent” enough to handle differences in lighting, depth, position of the sun, weather, etc. Doing this involves building more complex or sophisticated programs. Such programs almost always require significantly more computing hardware to execute and significant more capital investment in writing, testing and optimizing the program. All of these clearly increase costs.
The challenge is that it is basically impossible to see this from marketing demonstrations because from a demo all systems invariably look exactly alike. This of course has the vicious effect of encouraging people to choose cheaper systems that are more likely to generate high false alerts and be unmaintainable.
If you select a system that works, the cost per camera can make it difficult to justify the expense. Indeed, so much of the first generation video analytic deployments, came from government grant money, essentially making the cost secondary or not relevant. Nevertheless, for video analytics to grow in the private sector, they will not only need to work they will need to generate financial return.
When video analytics allow for guard reduction or reduce high value frequent losses, it is easy to justify and you see companies having success here (in terms of publicly documented cases, IoImage is the leader here). For other cases, where humans are not being eliminated, the individual loss is small or the occurrence of loss is low, the cost can be a major barrier.
Conclusion
Though I anticipate video analytics successes to increase, I believe such success will be constrained to applications where the loss characteristics and/or the human reduction costs are high. While analytics will certainly become cheaper, such cost decreases will take time and in the interim, it is these high value applications where analytics can gain a foothold of success.
Comparison Between IOimage and VideoIQ
http://spotonsecurity.com/2010/01/08/comparison-testing-is-hard-to-find/
By Posted by Doug Marman under VideoIQ 1/8/09
[6] Comments"I will try to be objective about the results, but of course that’s not as easy as it sounds.
The demos weren’t long. We each had about 10 minutes to show our products working. Not exactly an in-depth test. Both systems had to detect someone walking from behind the curtains across the room. With our system they also tested “Object Missing” and with IOimage they tested “Object Left Behind”.
Both products worked fairly well. Some of the differences were minor but interesting.
IOimage needed to calibrate their system before the event. They used two people and it took them about 30 minutes. However, since it was indoors, they could cut their process short. In general it followed the videos that they show on how to calibrate their products, but these guys were clearly experienced and didn’t have much time, so they moved fast. One person sat at the PC and marked the head and feet of the other person at four different places in the room. They drew a line on the floor, showing a predetermined distance (10′ in this case). They only calibrated one axis, not two. And they only calibrated in the one area that was going to be tested, not the whole room.
Our system doesn’t need calibration, as I’ve mentioned before. So, we had plenty of time. Picture the Maytag repair guy with his feet up, while waiting.
However, what we found interesting was the way IOimage positioned the whole process of calibration and tuning. They claimed calibration is what makes detection better. This is why they could detect someone crawling. However, as I’ve said before, this is wrong. While calibration clearly makes their system work better, in our case the calibration runs automatically. So, it is really a question of manual calibration compared to automatic calibration. As I’ve pointed out, there are lots of big disadvantages to manual calibration:
http://spotonsecurity.com/2009/11/05/the-tuning-and-calibration-controversy/
The issue about detecting crawling people is bogus. Perhaps they don’t realize it. They might think that calibration has something to do with this, but it doesn’t. It is simply that we have not yet developed an object classification type for people crawling. Quite frankly, we haven’t had any demand for it yet. The fact that we can still detect people crawling is easily demonstrated by setting our detection to “suspicious objects”, which easily captures people who crawl.
On the other hand, IOimage worked hard to make sure that they were getting a full view of the people in the area they wanted to detect, and they were especially concerned about moving chairs or tables out of the way. We didn’t have to worry about that, because we have an object classification type for head-and-shoulders detection of people. That’s pretty important indoors, because chairs and desks are quite common. IOimage apparently doesn’t have that object classification type, which is why they needed to see the whole person. In other words, this has nothing to do with calibration; it is all about the types of objects the system has been trained to detect.
You can read my previous post about the down side of manual calibration. But here are some other questions: How do you calibrate boats? Does someone have to walk out on the water? If you are setting up a system to watch the tarmac at an airport, do you have to shut the runway down while you walk out there to calibrate it? What about hazmat sites? Do you really need to send people in there to calibrate the camera? Besides all the other problems with having to manually calibrate a system, as I mentioned in my previous post, it isn’t always practical.
The real test comes down to how well do the systems work. That’s what really matters. Unfortunately, this wasn’t a stringent test. We wished it could have been outdoors with trees blowing in the background, to make it a tougher comparison. In general, both systems seemed to work well. We spotted some minor false detects on their system when the curtains moved, high up in the air where calibration should have ruled detection out, but for some reason didn’t.
Another noticeable difference was that they were only streaming the video from their camera, while our camera captures and records the event. Therefore, it appears to be more difficult with their system if you missed what just happened. In our system, you get a video clip that you can playback whenever you are ready. You don’t have to be there watching. IOimage would have to add an NVR to their system to get storage playback.
What IOimage added instead, that we don’t have, is mouse trails of where a person has been in the scene. This helps when it isn’t as easy to see where someone has been. You can just look at their previous trails. That works. I would rather watch the actual pre-alarm video so you can see what they were actually doing, but both systems offer something that works.
Apparently, from what we could see, IOimage can adjust sensitivity, but it seems to change it for the whole camera. We can individually set sensitivity for each detection rule. So, our missing object can be very sensitive, if we want, but people detection does not need to be.
One of the best things about their product is their web interface. Very well done. An integrator can do all of their calibration through the web, and it worked smoothly. If you have to calibrate, that’s a big plus.
However, they had to switch their camera from people detection to object left behind during the demo. Apparently it doesn’t detect both at the same time. Ours does, and in fact can run quite a few different types of detections with different types of objects, in different regions of interest, all at the same time on the same camera.
Also, once our rules are set up, our system keeps detecting even when you are changing or adding new rules. We were surprised to see that the IOimage system shuts down when you are setting up rules. It’s not a big issue. It is more important to detect a number of different things at the same time. That’s very useful.
It wasn’t much of a detailed competitive test, as I said. Both systems use high quality video analytics, not advanced video motion detection. And the results from both were pretty good.
And of course, this would be more objective if someone else were reporting it. However, it is so hard to find any comparison testing that I thought I would share it, anyway."
ObjectVideo's Poor Reputation and Blaming Others
http://ipvideomarket.info/report/objectvideo_poor_reputation_and_blaming_others
by John Honovich, IP Video Market Info posted on Feb 18, 2010 About John Contact John
The sad story of video analytics is now well known. Unfortunately, one of the ringleaders of this circus, ObjectVideo, has taken to blaming others while failing to prove their own effectiveness.
Poor Reputation
Video analytics suffers from an increasingly poor reputation, a hangover from the heady days of the mid 2000s. From my ongoing conversations with integrators and OEMs, ObjectVideo has a notably poor reputation, even relative to their peers. In ObjectVideo's defense, they were early, they were one of the heaviest marketers and they OEM their analytics to dozens of other companies.
Blaming Others
Twice in the last half year, ObjectVideo has pushed back on video analytic criticism, blaming others.
Last year, ObjectVideo called out the 'security industry', saying how they were tired of hearing how 'video analytics did not work.' ObjectVideo felt that a lack of training and optimization was at fault:
"Many in our industry correlate the need for trained users and the need to configure the analytics with the notion that analytics, as a whole, are immature and unreliable. Nothing could be further from the truth. This thinking highlights the resistance to change that exists within the security industry."
We believe it is the responsibility of manufacturers to make products work for the skill levels of integrators and the operational needs of end users. It is pointless to fight against this.
This month, ObjectVideo complained again about "hearing that analytics are 'still not ready for prime time.'” In this post, ObjectVideo emphasizes that other factors beyond the manufacturer's themselves contribute to analytic problems. It offer a long story about how a technicality cost them a project. In the comments, ObjectVideo agreed with our assertion that this was an outlier and not a common cause.
We believe it is misleading to make a big deal of a rare occurrence at the expense of addressing the main causes (i.e., the analytics themselves).
In defending themselves, ObjectVideo weakly asserted that, "In general, most [ObjectVideo] analytic solutions are deployed successfully." When we asked them what percentage were successful, they declined to answer. It leaves us wondering how many of their projects do fail and why they would offer a weak endorsement of their own solution.
Proving Their Worth
We would like to see ObjectVideo prove their worth and their viability. To that end, we have unsuccessfully asked for more technical information, case studies, etc over the past year with no positive outcome to date. ObjectVideo has very few public success stories nor technical information. They claim this is because their OEM practice restricts them from sharing such material. We think not only can they provide this but they need to do so to overcome their and their market's shaky reputation.
This being said, we are impressed that ObjectVideo has attempted to blog. Few companies in our market do so and even less actually speak their mind. The challenge we see is that rather than using the blog to build confidence and trust with the community, their repeated blaming and failure to speak at a substantive technical and operational level raises more issues. (By contrast, a good example that demonstrates technical expertise and builds trust is Genetec's blog. This is especially noteworthy since Genetec has historically been reluctant to publicly share information).
Video Analytics Market Future
We believe the best way for video analytics vendors to re-build their reputation is to prove clearly through real world successes and technical details that their products work well for real security integrators and users. Most are unlikely to believe hype and do not want finger pointing from the companies who funded the flood of unmet marketing claims.
Video Analytics Solutions Are Not All Created Equal
The use of video analytics is growing rapidly in the surveillance market. It has proven indispensable in high-risk security projects, and is becoming increasingly popular in commercial jobs for a wide range of applications, including outdoor protection, customer service measurements, people counting, crowd monitoring, and many others.
We are not far from the day when most video products will include embedded content analysis, making all video products smarter. However, there is a lack of solid technical information to help compare available technologies. The problem is that companies often make grand claims, but many fall far short in performance, leading to widespread disappointment.
The purpose of this article, therefore, is to outline the general principles of how video analytics works, in non-technical language, and examine how competing technologies try to solve these problems. The results vary dramatically, and a closer look shows why there are such big differences in performance.
Video analytics systems are built on three core components:
- Motion detection and object segmentation: This is where the video is processed to separate foreground objects from the background. This is the most processor intensive part of video analytics, accounting for up to 80% of the computational resources. However, there is a wide range in how well different products segment moving objects from the rest of the video.
- Object Tracking: This step tracks groups of pixels that are foreground objects as they move from frame to frame. If a group of pixels moves across the scene, it is probably a foreground object. The challenge is to track this blob of changing pixels. Once again, there is a huge range in performance from the different approaches taken.
- Object Classification: This function identifies the type of object detected. If a group of pixels moves across the scene, it is probably a foreground object. The challenge is to track this blob of changing pixels. Once again, there is a huge range in performance from the different approaches taken.
Systems vary in how well they perform each of these three steps. For example, there are many products being sold today as video analytics that are better described as â€Å“advanced video motion detection.†They can extract blobs of pixels moving across a scene, but cannot tell whether these objects are people, vehicles or anything else. In other words, they have no object classification.
Such systems have a harder time distinguishing a branch blowing in the wind from a person, but some of them have added object size calibration to help ignore things that are too big or too small. Large animals and tree branches are still a problem with these technologies, and they also require someone manually calibrating each camera for object sizes. Advanced video motion detection systems might be able to perform all the behavior detection types you see in the best video analytics systems, such as tripwires, direction of travel, intrusion detection, etc., but their results cannot compare to the accuracy of systems with superior object classification.
This is just one example of where some products skimp to reduce the amount of processing required. The problem is that you can’t easily tell the difference by just looking at such solutions or reading their literature. They claim to offer all of the same features you see in high end systems. However, the differences in results are stark.
Even the best of the advanced video motion detection systems are at least 10X worse compared to even the poorest true video analytics products. Extensive testing easily proves this out, but most integrators and users don’t have the time to test dozens of products to find one that works.
For a more detailed description of how video analytics works, read All Analytics are not Created Equal by Doug Marman, CTO and VP of Products at VideoIQ, at http://www.videoiq.com/products/resource-center.
VideoIQ's Video Analytics Testing
Challenges with Analytics
Over the last few years, analytic performance has been a hotly debated topic with many users finding disappointing results.
Increasing the challenge was a lack of public independent test results. With huge marketing campaigns but minimal 3rd party technical reviews available, determining what worked and how it worked were hard questions to answer.
Summary of VideoIQ Analytic Test
These are key findings from our test:
- False Positives in a variety of environments were uncommon. A small number of conditions generated periodic false positives.
- False Negatives (missing suspects) was generally very low except for a number of conditions that should be carefully considered.
- Performance was achieved without any software configuration; However, users should recognize system limitations and plan accordingly.
- Initial start-up and system re-starts created temporary reductions in performance
- Performance was consistent with technical guidelines provided by VideoIQ. However, some of the more aggressive marketing claims were not consistent with our test results.
Friday, February 26, 2010
Intel may acquire FPGA vendor Xilinx or Altera
EE Times
(01/13/2010 12:57 PM EST)
Christopher Danely, an analyst with JP Morgan Securities Inc., said in a report titled "Top 10 Semiconductor Predictions for 2010" that the year could bring a big strategic acquisition to the semiconductor space. In addition to Intel acquiring an FPGA vendor, Danely speculated that other large acquisitions could be possible, including Analog Devices Inc. (ADI) buying a power management IC company and Microchip Technology Inc. buying a company to expand its presence in the microcontroller market.
Intel has been gearing up to expand its presence in the embedded space, last year acquiring embedded software specialist Wind River Systems Inc. andporting unspecified Atom processor cores to Taiwan Semiconductor Manufacturing Co. Ltd. (TSMC)'s technology platform. Danely speculates that an acquisition of Xilinx or Altera would jump start these efforts, but it is unclear how the FPGA technologies would mesh with Intel's. Like many companies, Intel previously played in the programmable logic space but abandoned those efforts years ago.
Danely said that both Actel Corp. and Lattice Semiconductor Corp. would be unlikely targets for Intel (Santa Clara, Calif.) because of their relatively small size. Xiliinx (San Jose, Calif.), the market leader in programmable logic, would make a more attractive target for Intel than No. 2 player Altera (San Jose), Danely wrote, because Xilinx has higher operating expenses and could offer Intel higher cost savings opportunities.
Altera, a customer of Taiwan Semiconductor Manufacturing Co. (TSMC), has in recent years maintained a process technology migration lead over Xilinx, which uses foundry suppliers United Microelectronics Corp. (UMC), Samsung Electronics Co. Ltd. and others. Danely speculated that Intel could significantly boost Xilinx by buying the company and migrating its products to Intel process technology, which is the most advanced in the industry.
Another Wall Street analyst, Hans Mosesmann of Raymond James Equity Research, doesn't share Danely's view. Mosesmann said his firm would "wholeheartedly disagree" with speculation that Intel might buy Xilinx or Altera, at least in 2010.
Mosesmann said Intel really wants to be relevant in wireless and smartphones. An acquisition of Xilinx or Altera would have no value in that endeavor, he said.
Mosesmann noted that Intel already tried its hand in programmable logic but ultimately sold its PLD business to Altera for about $50 million in 1994. "They've been there," he said. "They've done that. It's not their gig."
Intel, which has about $13 billion in cash on its balance sheet, would certainly have to dig deep to buy Xilinx. Xilinx has a market capitalization of roughly $6.7 billion, and shareholders would no doubt expect a premium over the company's current stock price of a little over $24.
Other acquisition scenarios, predictions
In other potential semiconductor acquisition scenarios, Danely speculated that Monolithic Power Systems Inc., Intersil Corp. and National Semiconductor Corp. could be strategically attractive to ADI since all three have the majority of their product portfolios in power management, where ADI has a limited presence despite investing heavily in over the past few years.Danely also speculated that Microchip could make another bid to acquire Atmel Corp. to supplement its microcontroller business. Although Microchip'sprevious bid to acquire Atmel failed in February 2009, "we believe both companies could revisit the a deal if they can could work out an agreement on how to divest [Atmel's] other lines of business," Danely wrote.
In other predictions, J.P. Morgan expects Xilinx to continue to gain market share at the expense of Altera due to Xilinx' presumed lead at 65-nm, Danely wrote. But the firm expects Altera to gain back share in the second half of the year thanks to its lead in the 40-nm high density category with the Stratix IV family.
Danely also predicted that semiconductor capital expenditures in 2010 would disappoint bulls who see a big year for equipment suppliers. "While we agree capacity is tight, we believe the problem is mostly back -end- related," Danely said. "We would note back-end capacity additions do not cost as much as front-end capacity additions."
Thursday, February 25, 2010
Cablevision TV-PC media relay service
BETHPAGE, N.Y., Feb. 24 /PRNewswire-FirstCall/ -- Cablevision Systems Corp. (NYSE: CVC) today announced the development of a new, first-of-its-kind service, called PC to TV Media Relay, that will allow its digital cable customers to relay whatever information or images currently appear on their computer screen to their television in real-time, without any additional equipment in the home, using only the company's advanced fiber-rich network.
This innovative service will allow Cablevision customers, with the press of a button, to transfer anything available for display on their PC, whether the information is stored on their PC, transferred from a drive or accessible on the Web, to the television for viewing on a dedicated channel that is accessible only by that customer. Cablevision plans to begin a technical trial of PC to TV Media Relay for the PC by June 2010.
"With our PC to TV Media Relay service, we are putting an end to the need for families to huddle around their laptops or PCs to watch content together. This new service will make it easy for our television customers to take broadband services including Internet video, as well as family photos or anything else displayed on a computer screen and move it to the television with the click of the mouse," said Tom Rutledge, Cablevision's Chief Operating Officer. "Cablevision has always provided our customers with advanced and easy-to-use services that leverage the power of our fiber-rich network to deliver the best possible experience."
Specific examples of the kind of content that consumers currently view on their PC, and will now be viewable on the television include:
- Personal stored media such as photos, home videos and music;
- Internet content including streaming video sites and audio such as Internet radio;
- Some productivity applications including email, documents and spreadsheets;
- And, other Desktop applications such as widgets.
The service will enable the customer to securely send information on their PC in real-time through Cablevision's network facilities to a dedicated channel viewable only by that customer. The service will completely eliminate the need to change input settings on the TV, as is the case with most in-home networking alternatives, or to purchase and install expensive additional equipment. A simple one-time software download to the computer will enable the PC to TV Media Relay service.
The technology that enables PC to TV Media Relay may also be extended to other consumer devices in the home including handheld devices running applications and connected to in-home wireless networks. PC to TV Media Relay for Mac is also in development.
Sunday, February 21, 2010
Saturday, February 20, 2010
3U VPX Xilinx® FPGA Processor Board
Leesburg, VA -May 11, 2009 -- Curtiss-Wright Controls Embedded Computing, a leading designer and manufacturer of commercial off-the-shelf (COTS) VME, VPX, VXS, and CompactPCI products for the aerospace and defense (A&D) market has announced a flexible, multi-card 3U VPX solution for rugged deployed embedded FPGA processing applications. With the interoperability of its 3U VPX FPE320 FPGA processor card, 3U VPX VPX3-450 FPGA processor and 3U VPX VPX3-127 single board computer (SBC) running Wind River's VxWorks, Curtiss-Wright Controls delivers an unmatched level of flexibility for addressing high-performance 3U VPX systems applications.
"Space, weight and power (SWaP) requirements are driving customers to use the largest FPGAs in their systems, while still needing general purpose processing and I/O. The FPE320/VPX3-450/VPX3-127 platform provides both in a rugged 3U form factor," said Lynn Patterson, vice president and general manager of Modular Solutions, Curtiss- Wright Controls Embedded Computing.
Using this solution, system integrators can now utilize the largest Xilinx Virtex-5 FPGAs currently available in a small form factor embedded system powered with a high performance Power Architecture general purpose processor. The FPE320 supports
Virtex-5 LXT, SXT, or FXT devices. With its onboard FMC expansion site (FPGA Mezzanine Card: ANSI/VITA 57) the FPE320 supports optimized FPGA I/O using Curtiss-Wright's broad range of FMC ADC51x series cards to bring in high speed I/O such as analog to digital converters (ADCs). The VPX3-450 FPGA processor combines the computing power of a Xilinx Virtex-5 FPGA with the high-performance floating-point capabilities of the Freescale 8640D dual-core Power Architecture processor. The VPX3-127 features a Freescale MPD8640D processor and can be expanded via its onboard PMC/XMC expansion site. The VPX3-127 uses PCI Express to connect to the FPE320 and/or to the VX. All three boards are available in a combined development chassis that includes FPE320 and/or VPX3-450 cards and a VPX3-127 card.
Systems designers can use the platform for prototyping before addressing extended temperature convection and conduction-cooled systems.
About the FPE320 VPX Virtex-5 FPGA Card
The FPE320 is a 3U VPX FPGA processor board that incorporates the largest Xilinx Virtex-5 FPGAs available with an FMC mezzanine site. Providing a large amount of resources in a small, rugged form factor, the FPE320 is the ideal FPGA platform for 3U systems that need to acquire analog and other high-speed I/O or need a large FPGA processor.
FPE320 Features:
* Xilinx Virtex-5 3U VPX processor card with FMC Site
* Supports Xilinx Virtex-5 SX240T, LX220T/LX330T, and FX200T FPGAs
* FMC (VITA 57) mezzanine site for I/O
DDR2 SDRAM and QDRII SRAM memory resources
* Four x4 high-speed serial interconnects to the backplane for PCI Express, Aurora or Serial RapidIO
* Additional low-speed I/Os to the backplane
* FusionXF Development Kit for HDL development
* VxWorks 6.x and Linux Support
* 3U VPX with .8" Pitch
* Air and conduction cooled options
About the VPX3-450 FPGA Processor Card
The VPX3-450 is a 3U VPX FPGA processor board that incorporates the largest Xilinx Virtex-5 FPGAs available with an on-board PMC/XMC mezzanine site. With large DDR2 SDRAM and fast QDR-II+ SRAM blocks, and several on-board and off-board high-speed serial links, the VXP3-450 FPGA node provide a balanced mix of processing capabilities with memory, inter-FPGA, and off-board bandwidths.
VPX3-450 Features:
* 3U VPX-REDI
* One Xilinx Virtex-5 Platform FPGA (LX155T, or SX95T)
* 512 Mbytes DDR2 SDRAM attached to the FPGA, 2.2 GBytes/sec peabandwidth
* 18 Mbytes QDR-II+ SRAM attached to the FPGA in two banks, 4.4 Gbytes/sec peak bandwidth per FPGA
* 1 GHz 8640D dual-core processor with 1 Gbytes of DDR2 SDRAM in two banks
* XMC mezzanine site
* On-board PCI Express switch with 4-lane connectivity to the PowerPC, the FPGA, the mezzanine site, and a 4-lane port to the backplane
* One additional 4-lane backplane fabric port to the PowerPC. May be either PCI Express or Serial RapidIO
* Two 4-lane MGT ports to the backplane
* 256 Mbytes Flash for PowerPC or FPGA code or user files
* Support for ChipScope Pro and JTAG processor debug interfaces
* Continuum FXtools developer's kit offers Continuum Firmware and BSP, 450-specific software libraries, BIT, highly-optimized IP blocks, reference designs, and a scriptable simulation testbench
* Low and high temperature-qualified IP blocks
* Air-cooled and conduction-cooled versions
About the VPX3-127
The 3U VPX VPX3-127 single board computer combines the performance and the advanced I/O capabilities of Freescale's MPD8640D Power Architecture processor with an extensive I/O complement to provide a highly capable processing platform for a wide range of embedded military/aerospace applications. Designed for space, weight and power-constrained applications, the VPX3-127 represents the latest step in the evolution of rugged high-performance, highly integrated small form factor single board computers.
VPX3-127 Features:
* Powerful general-purpose single board computer with Freescale MPD8640D
* Dual Freescale Power Architecture cores up to 1.0 GHz
* Up to 2 GB DDR2 SDRAM controlled by dual 64-bit controllers
* Full complement of I/O capability (Ethernet, serial, USB 2.0, PCI Express, TTL and differential discretes.)
* VPX format with two 4-lane PCI Express fabric ports or one 4-lane PCI Express port and one 4-lane SRIO port
* VxWorks 6.x BSP, Driver Suite supporting Workbench 2.x IDE, and Curtiss-Wright Wind River Linux GPP LE
* Continuum Software Architecture (CSA) firmware providing a comprehensive suite of system debug, exerciser, and update functions, BIT, and non-volatile memory sanitization function
* Designed for military harsh-environment applications, both air- and conductioncooled
The FPE320, VPX3-450, and VPX3-127 are available for development systems in 8 weeks ARO. All of these boards are available in both commercial and rugged versions. For more information on these products click on the appropriate links - FPE320, VPX3-450 and VPX3-127.
Friday, February 19, 2010
Video Quality Measurement Metrics
Video quality measurement has been gaining more attention. Video service providers, television network operators, surveillance equipment manufacturers and broadcasters need to ensure that the video products and services they offer meet minimum quality requirements. Although the network bandwidth has been increased but higher and higher resolution of video is being carried over limited bandwidths. There are several video quality metrics including PSNR, VQM, MPQM, SSIM and NQM.
PSNR
PSNR is derived by setting the mean squared error (MSE) in relation to the maximum possible value of the luminance as follows:
Where f(i,j) is the original signal at pixel (i, j), F(i, j) is the reconstructed signal, and M x N is the picture size. The result is a single number in decibels, ranging from 30 to 40 for medium to high quality video.
Despite several objective video quality models have been developed in the past two decades, PSNR continues to be the most popular evaluation of the quality difference among pictures.
Video Quality Metric (VQM)
VQM is developed by ITS to provide an objective measurement for perceived video quality. It measures the perceptual effects of video impairments including blurring, jerky/unnatural motion, global noise, block distortion and color distortion, and combines them into a single metric. The testing results show VQM has a high correlation with subjective video quality assessment and has been adopted by ANSI as an objective video quality standard.
Moving Pictures Quality Metric (MPQM)
MPQM is an objective quality metric for moving picture which incorporates two human vision characteristics as mentioned above. It first decomposes an original sequence and a distorted version of it into perceptual channels. A channel-based distortion measure is then computed, accounting for contrast sensitivity and masking. Finally, the data is pooled over all the channels to compute the quality rating which is then scaled from 1 to 5 (from bad to excellent).
The actual computation of the distortion E for a given block is given by

where









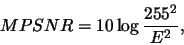
or can be mapped to the 5-point ITU quality scale using the equation
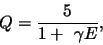
where




Structural Similarity Index (SSIM)
SSIM uses the structural distortion measurement since the human vision system is highly specialized in extracting structural information from the viewing field and it is not specialized in extracting the errors.
If you let x = {xi | i = 1,2,…,N} be the original signal and y = {yi | i = 1,2,…,N} be the distorted signal the structural similarity index can be calculated as:
with
-
the average of
;
-
the average of
;
-
the variance of
;
-
the variance of
;
-
the covariance of
and
;
-
,
two variables to stabilize the division with weak denominator;
-
the dynamic range of the pixel-values (typically this is
);
-
and
by default.
Noise Quality Measure (NQM)
In NQM, a degraded image is modeled as an original image that has been modeled as an original image that has been subjective to linear frequency distortion and additive noise injection. These two sources of degradation are considered independent and are decoupled into two quality measures: a distortion measure (DM) of the effect of frequency distortion, and a noise quality measure (NQM) of the effect of additive noise. The NQM takes into account : (1) variation in contrast sensitivity with distance, image dimensions; (2) variation in the local luminance mean; (3) contrast interaction between spatial frequencies; (4) contrast masking effects. The DM is computed in three steps. First, the frequency distortion in the degraded image is found. Second, the deviation of this frequency distortion from an all-pass response unity gain is computed. Finally, the deviation is weighted by a model of the frequency response of the human visual system.
Followers
Blog Archive
-
▼
2010
(99)
-
▼
February
(18)
- Deinterlacing
- Video Analytics Survey
- Comparison Between IOimage and VideoIQ
- ObjectVideo's Poor Reputation and Blaming Others
- Video Analytics Solutions Are Not All Created Equal
- VideoIQ's Video Analytics Testing
- Intel may acquire FPGA vendor Xilinx or Altera
- Cablevision TV-PC media relay service
- The Fastest Soft H.264 Decoder
- 3U VPX Xilinx® FPGA Processor Board
- Video Quality Measurement Metrics
- TI DM368
- TI HD 1080p Digital Media Roadmap
- Panasonic 3D Camcorder
- 3D DIGITAL PRINTING
- Apple patent may be clue to '3D' tablet control
- 3D Soccer Match
- Altera Unveils Innovations for 28-nm FPGAs
-
▼
February
(18)